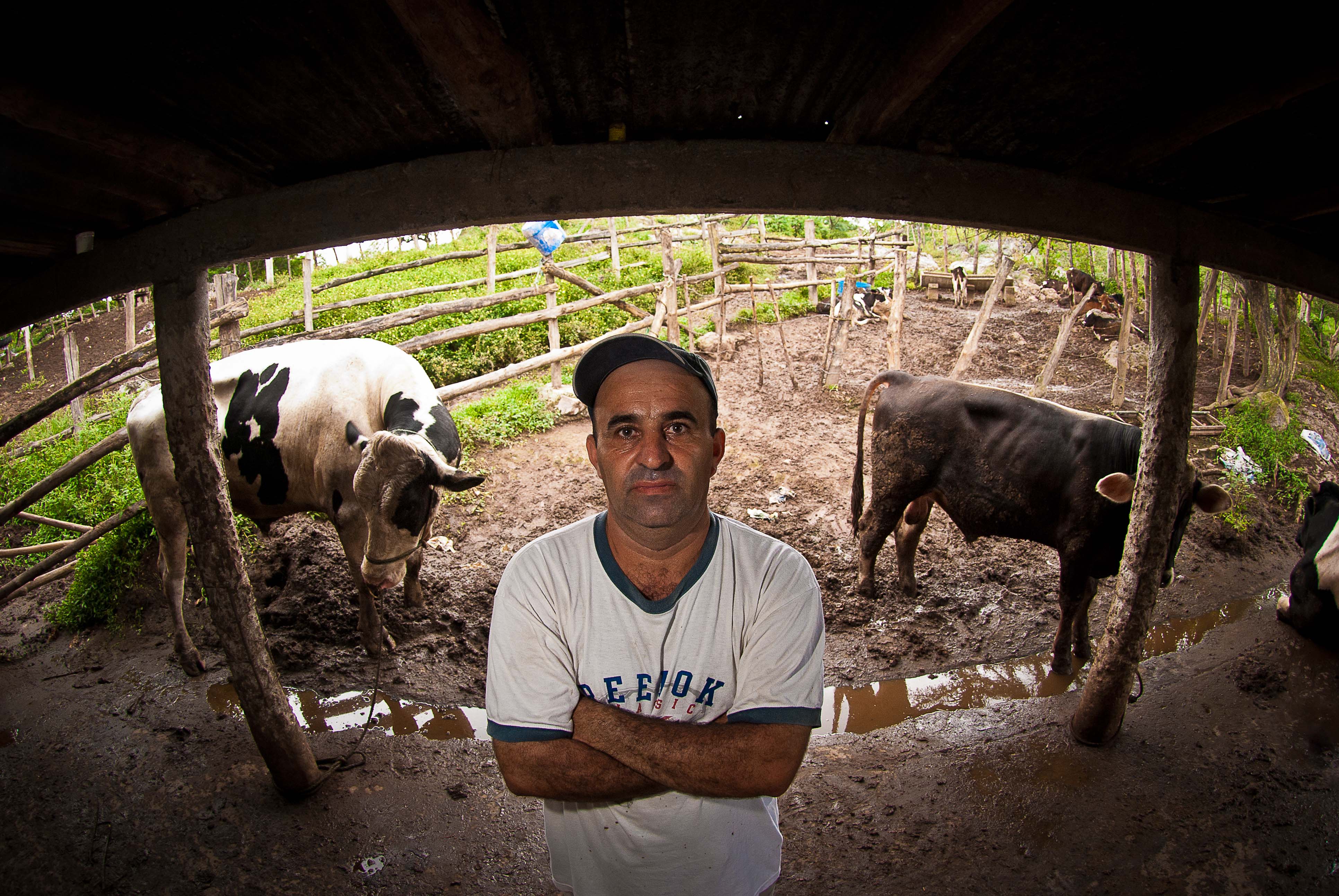
MCC released impact evaluations of farmer training activities in five countries in October 2012. Looking across these five—and informed by lessons about designing and implementing impact evaluations in agriculture more broadly—MCC identified ways to improve the design of agriculture projects and how to evaluate them. In addition to improving our own practices, we hope other development organizations can use what we’ve learned to plan their own programs and evaluations.
- Test traditional assumptions and build in ways to compare different types or levels of interventions. In most MCC agriculture projects so far, the treatment groups received a similar level or type of training or assistance, so impact evaluations could only compare the impact of the intervention to no intervention. In many cases, it might be more interesting to compare several variations of the intervention to test which ones are more effective at delivering results. It is sometimes difficult to build these variations into a project, and if it isn’t done in the beginning, it is much harder to do so later and practically impossible once implementation begins.
Currently, MCC and MCAs are exploring using impact evaluations to test assumptions around the appropriate content and duration of training to maximize impact. However, this is challenging and might mean managing different contracts for different activities instead of one contract for all planned training —leading to a loss of economies of scale in some cases. In addition, with more categories of treatment groups, the more difficult it is to have sufficient statistical power in the sample to compare across groups. As with all evaluation efforts, this requires close coordination between the sector experts responsible for designing the projects and the monitoring and evaluation experts responsible for developing the evaluations. - The randomized roll-out evaluation approach has risks. In a randomized roll-out, a first round treatment group is compared to a second round treatment group that receives the intervention at a later date. The upside is that all eligible groups, assigned to different phases of the project, will receive the intervention. The key to this approach is that enough time passes between the two phases to see changes in behavior and an accruing of benefits for the first group before the second group participates.
In many MCC projects, however, delays occur in the first round. Then, the second round receives the intervention according to the roll-out timeframe—but before enough time has passed to really see impacts. As a result, learning using these evaluations is more limited. Randomized roll-out is only appropriate when both detectable changes are expected very soon after the intervention and the timing of rolling out the intervention to the second group follows the program logic and time needed to see and measure impacts. - Learning from evaluations is limited when an activity’s objective, logic, participants, and expected results have not been clearly defined in the first place. While these elements are always stated in some form, the definitions are often not clear up-front or shared by all stakeholders. As a result, the interpretation of this language may allow for great variance in the size and range of the investments, the type or length of training offered and the key criteria for selecting participants. All these uncertainties make projects difficult to plan and implement and also make rigorous impact evaluations even more difficult. MCC is committed to improving the quality and documentation of project design so that it can better inform project implementation and evaluation.
- Calculations to determine statistical significance are important. The number of observations, the diversity of beneficiaries, the primary outcomes selected, and the expected treatment effect on those outcomes all feed into our level of certainty about whether results are based on the MCC-funded project, and not something random. If the number of people or groups is too low or too diverse, or how much the investments are expected to help is relatively small, then an impact evaluation may not be able to report statistically significant results. In designing the impact evaluation, it is best to be conservative, so that as facets of the program change or data collection turns out to be more difficult than planned, enough statistical significance remains to produce meaningful results. It is important to conduct calculations as early as possible so that the evaluation design can be changed or the evaluation cancelled before too many resources are wasted on evaluations that are unable to report on the most important indicators.
We want to hear from you! Do you have any experiences and lessons from evaluating agriculture projects?